Keyword AI dala – AI dala sets the stage for an exploration of artificial intelligence’s transformative role in data processing. This journey delves into the multifaceted meanings of “AI dala,” examining its implications across various industries and cultures. We’ll analyze how AI algorithms process vast datasets, highlighting both their remarkable capabilities and inherent limitations. Ethical considerations, future trends, and illustrative examples will illuminate the complex interplay between AI and data in shaping our world.
From understanding the nuances of the term “AI dala” itself to predicting the future of AI-driven data analysis, we’ll cover a wide spectrum of topics. We’ll explore the ethical dilemmas inherent in using AI for data analysis, the importance of data privacy, and the need for responsible AI development. Through hypothetical scenarios and real-world examples, we aim to provide a comprehensive overview of this rapidly evolving field.
Daftar Isi :
Understanding “AI dala”: Keyword AI Dala
The phrase “AI dala” appears to be a neologism, a newly coined word or phrase, likely combining aspects of artificial intelligence (“AI”) and an unclear second element, “dala.” Its meaning and implications are highly dependent on the intended context and the interpretation of “dala.” Without further information on its origin or intended use, we can only speculate on its possible meanings.The potential meanings of “AI dala” could range from technical to cultural.
It might refer to AI-driven data analysis, AI-powered data manipulation, or even a specific type of AI system or algorithm. The word “dala” itself might be a term from a specific language or field, or it could be a deliberate misspelling or creative alteration of an existing word. Further analysis is needed to fully understand its intended significance.
Potential Interpretations of “AI dala”
Several interpretations of “AI dala” are possible depending on the context. It could be a portmanteau, combining elements of “AI” and a word related to data or information. For example, if “dala” is meant to evoke the idea of “data,” then “AI dala” could refer to AI systems that process and analyze large datasets. Alternatively, if “dala” is intended as a shortened or modified form of a word meaning “flow” or “stream,” then “AI dala” could describe a continuous stream of data processed by AI.
Another possibility is that “dala” is a word from a non-English language with a relevant meaning, requiring further linguistic investigation to determine its exact contribution to the phrase.
Examples of “AI dala” in Various Industries
Hypothetically, “AI dala” could find applications in several industries. In finance, it could represent an AI system used for real-time fraud detection by analyzing financial transaction data. In healthcare, it could refer to AI algorithms processing patient data to predict disease outbreaks or personalize treatment plans. In marketing, it might describe an AI-powered system that analyzes consumer data to optimize advertising campaigns.
These are merely speculative examples; the actual meaning and usage of “AI dala” would need to be clarified by its creators or users.
Cultural and Regional Nuances of “AI dala”
The cultural or regional nuances of “AI dala” are highly uncertain without knowing its origin. If “dala” is a word from a specific language or dialect, the phrase could carry significant cultural weight and implications depending on the word’s meaning and cultural associations within that language. For example, if “dala” has positive connotations related to knowledge or wisdom, then “AI dala” might be perceived positively.
Conversely, if it has negative connotations, the phrase might be viewed with skepticism or distrust. Further research into the possible linguistic origins of “dala” is necessary to explore this aspect further.
AI’s Role in Data Processing
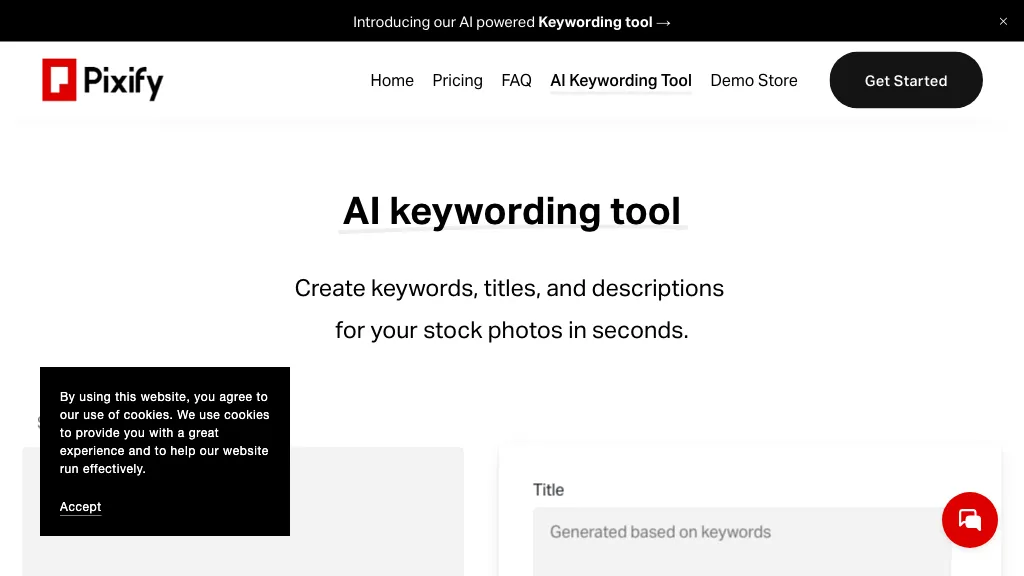
Source: topai.tools
AI is revolutionizing data processing, enabling us to handle and analyze data volumes previously unimaginable. Its ability to learn patterns, make predictions, and automate tasks makes it an invaluable tool across numerous industries. This section explores how AI processes data, the algorithms involved, and the challenges it faces.AI employs various techniques to process and analyze large datasets. These techniques leverage the power of machine learning and deep learning algorithms to extract meaningful insights from raw data.
The process often involves several steps, including data cleaning, transformation, feature engineering, model training, and evaluation.
Keyword AI dala is a broad term encompassing various applications of artificial intelligence. A significant part of this involves the development of advanced conversational interfaces, like the sophisticated AI chatbots that are transforming how we interact with technology. Understanding the nuances of Keyword AI dala requires exploring these chatbot advancements and their impact on communication and information retrieval.
AI Algorithms for Data Processing
Different AI algorithms are suited for different types of data and tasks. For example, supervised learning algorithms, such as linear regression and support vector machines (SVMs), are effective for prediction tasks where labeled data is available. Unsupervised learning algorithms, like k-means clustering and principal component analysis (PCA), are used to discover patterns and structures in unlabeled data. Deep learning algorithms, including convolutional neural networks (CNNs) and recurrent neural networks (RNNs), excel at handling complex, high-dimensional data, often used in image recognition, natural language processing, and time series analysis.
The choice of algorithm depends heavily on the specific problem and the characteristics of the data.
The keyword “AI dala” suggests a focus on data’s role in artificial intelligence. Understanding how AI arrives at its conclusions is crucial, which is where the concept of Explainable AI comes in. To learn more about making AI’s decision-making processes transparent, check out this resource on Explainable AI. Ultimately, understanding Explainable AI helps refine the use of “AI dala” and improves the overall effectiveness of AI systems.
Challenges and Limitations of AI in Data Processing
Despite its capabilities, AI faces challenges in handling complex data. One significant challenge is the need for large, high-quality datasets for training effective models. Insufficient or biased data can lead to inaccurate or unfair results. Another challenge is the computational cost associated with training complex AI models, particularly deep learning models. These models require significant computing power and time to train, which can be a barrier for organizations with limited resources.
Furthermore, interpreting the results of complex AI models can be difficult, making it challenging to understand the reasoning behind the model’s predictions. Finally, ensuring data privacy and security is crucial when dealing with sensitive information, posing additional complexities for AI-driven data processing.
Hypothetical Scenario: AI in Efficient Data Management
Imagine a large e-commerce company with millions of customer transactions daily. Manually analyzing this data to identify trends, predict customer behavior, and personalize marketing campaigns would be incredibly time-consuming and inefficient. However, by employing AI-powered data processing, the company can automate several tasks. AI algorithms can analyze past purchase history, browsing behavior, and demographic information to identify customer segments with similar preferences.
Keyword AI dala is a broad term encompassing many applications of artificial intelligence. One exciting area where AI is making huge strides is in gaming, as you can see from this article on AI in gaming. The advancements in AI within game development directly impact the capabilities and complexity of games, ultimately feeding back into the evolution of AI dala applications as a whole.
Therefore, understanding AI in gaming helps broaden the understanding of Keyword AI dala.
This allows for targeted marketing campaigns, increasing conversion rates and customer satisfaction. Furthermore, AI can predict future demand for products, optimizing inventory management and reducing storage costs. AI-powered fraud detection systems can identify suspicious transactions in real-time, minimizing financial losses. In this scenario, AI significantly improves efficiency, reduces operational costs, and enhances decision-making.
Ethical Considerations of AI and Data
The increasing reliance on AI for data analysis brings forth a complex web of ethical considerations. The power to process vast amounts of data quickly and efficiently also presents significant risks if not handled responsibly. Understanding and mitigating these risks is crucial for ensuring the beneficial and equitable application of AI.
Data Privacy and Security, Keyword AI dala
Data privacy and security are paramount in the age of AI. The very nature of AI algorithms, which often require large datasets for training and operation, necessitates robust security measures to prevent unauthorized access, misuse, and breaches. A data breach involving sensitive personal information can have devastating consequences for individuals, leading to identity theft, financial loss, and reputational damage.
Furthermore, the use of AI in surveillance technologies raises concerns about potential abuses of power and erosion of individual liberties. Strong encryption, access control mechanisms, and anonymization techniques are essential for protecting sensitive data used in AI systems. Regular security audits and compliance with relevant data protection regulations are also crucial.
Algorithmic Bias and Fairness
AI algorithms are trained on data, and if that data reflects existing societal biases, the resulting AI system will likely perpetuate and even amplify those biases. This can lead to unfair or discriminatory outcomes in areas like loan applications, hiring processes, and even criminal justice. For example, a facial recognition system trained primarily on images of light-skinned individuals may perform poorly when identifying individuals with darker skin tones, leading to misidentification and potentially harmful consequences.
Addressing algorithmic bias requires careful data curation, algorithm design that explicitly accounts for fairness, and ongoing monitoring of AI systems for bias.
Transparency and Explainability
Many AI systems, particularly deep learning models, are often described as “black boxes” due to their complexity and opacity. Understanding how these systems arrive at their decisions is crucial for building trust and accountability. Lack of transparency can make it difficult to identify and correct errors, biases, or unintended consequences. The development of explainable AI (XAI) techniques aims to address this challenge by providing insights into the decision-making processes of AI systems.
This increased transparency allows for better understanding, improved debugging, and increased user trust.
Accountability and Responsibility
Determining accountability when an AI system makes a mistake or causes harm is a significant ethical challenge. Is the developer, the user, or the AI itself responsible? Establishing clear lines of responsibility is crucial for ensuring that AI systems are used responsibly and that appropriate redress is available in case of harm. This requires careful consideration of legal and regulatory frameworks, as well as the development of ethical guidelines and best practices for AI development and deployment.
Ethical Frameworks for AI and Data
Understanding the ethical implications of AI requires considering various ethical frameworks. The following table compares several prominent frameworks:
Ethical Framework | Key Principle | Application to AI | Potential Challenges |
---|---|---|---|
Utilitarianism | Maximize overall well-being | AI systems should be designed to produce the greatest good for the greatest number of people. | Difficult to predict and measure long-term consequences; potential for sacrificing individual rights for the collective good. |
Deontology | Adherence to moral duties and rules | AI systems should be programmed to respect fundamental rights and avoid actions that are inherently wrong, regardless of the consequences. | Defining universal moral rules can be challenging; conflicts may arise between different duties. |
Virtue Ethics | Cultivating virtuous character traits | AI systems should be designed and used in a way that reflects virtues such as fairness, honesty, and responsibility. | Subjectivity in defining virtues; difficulty in translating virtues into concrete design principles. |
Rights-Based Ethics | Protection of individual rights | AI systems should respect and protect individual rights such as privacy, autonomy, and freedom from discrimination. | Balancing individual rights with collective interests; defining the scope of relevant rights in the context of AI. |
Best Practices for Responsible AI Development and Data Handling
Responsible AI development requires a proactive approach to ethical considerations throughout the entire lifecycle of an AI system. This includes:
Prioritizing ethical considerations from the initial design phase through deployment and ongoing monitoring is crucial. This involves:
- Data Governance: Establishing clear policies and procedures for data collection, storage, use, and disposal, ensuring compliance with relevant regulations (e.g., GDPR, CCPA).
- Bias Mitigation: Implementing techniques to identify and mitigate bias in data and algorithms.
- Transparency and Explainability: Designing AI systems that are transparent and explainable, allowing users to understand how decisions are made.
- Accountability Mechanisms: Establishing clear lines of responsibility for the actions of AI systems.
- Continuous Monitoring and Evaluation: Regularly monitoring and evaluating AI systems for unintended consequences and biases.
- Human Oversight: Maintaining appropriate levels of human oversight to ensure responsible use of AI.
- Ethical Review Boards: Establishing ethical review boards to assess the ethical implications of AI projects before deployment.
The Future of AI and Data
The intersection of artificial intelligence and data is rapidly evolving, promising a future where data management and analysis are fundamentally reshaped. Advancements in AI are not just incremental improvements; they represent a paradigm shift in how we collect, process, and utilize information, leading to both unprecedented opportunities and significant challenges.The next decade will witness a dramatic acceleration in AI’s impact on data strategies.
This isn’t merely about faster processing speeds; it’s about a fundamental change in how we approach data itself.
AI-Driven Data Management Strategies
AI will increasingly automate various aspects of data management, from data cleaning and integration to anomaly detection and predictive maintenance. For example, AI-powered systems can automatically identify and correct inconsistencies in datasets, significantly reducing the time and resources currently dedicated to manual data cleansing. Furthermore, AI algorithms can proactively identify potential data breaches or security vulnerabilities, improving overall data security and integrity.
This proactive approach, driven by machine learning, will move data management from a reactive to a predictive model.
Breakthroughs in AI-Driven Data Analysis
We can anticipate several key breakthroughs in AI-driven data analysis over the next decade. One likely development is the widespread adoption of explainable AI (XAI), which will enhance the transparency and interpretability of AI-driven insights. This will be crucial in building trust and ensuring accountability in high-stakes applications, such as medical diagnosis or financial risk assessment. Another significant advance will be in the area of federated learning, allowing AI models to be trained on decentralized datasets without compromising data privacy.
This will unlock the potential of massive, distributed datasets without the need for centralized data repositories, a crucial development for sensitive data like medical records or personal financial information. Finally, we can expect to see significant progress in the development of AI systems capable of handling increasingly complex and unstructured data, such as images, videos, and natural language text, unlocking valuable insights currently inaccessible through traditional methods.
For example, AI could analyze medical images with greater accuracy and speed than human radiologists, leading to earlier and more effective diagnoses.
Societal Impacts of Increased AI Reliance on Data
The increased reliance on AI and data will have profound societal impacts. On the positive side, AI can drive improvements in healthcare, education, and environmental sustainability. AI-powered diagnostic tools can lead to earlier disease detection and personalized treatment plans. AI tutors can provide customized learning experiences, catering to individual student needs. AI-driven optimization can improve resource allocation and reduce environmental waste.
However, there are potential downsides. Increased reliance on AI for decision-making could exacerbate existing biases present in data, leading to unfair or discriminatory outcomes. The potential for job displacement due to automation is also a significant concern, requiring proactive measures to reskill and upskill the workforce. Moreover, the concentration of data in the hands of a few powerful tech companies raises concerns about privacy, transparency, and accountability.
The misuse of AI-powered surveillance technologies also poses a threat to civil liberties. The example of facial recognition technology being used for mass surveillance highlights these concerns.
Adaptable Regulations for AI and Data
Given the rapid pace of technological advancement, adaptable regulations are crucial to ensure the responsible development and deployment of AI and data technologies. Rigid regulations risk stifling innovation, while a lack of regulation could lead to unintended negative consequences. A flexible regulatory framework should prioritize ethical considerations, data privacy, and accountability. This framework should be designed to evolve alongside technological advancements, ensuring it remains relevant and effective in addressing emerging challenges.
The regulatory landscape needs to foster collaboration between governments, industry, and civil society to create a responsible and equitable AI ecosystem. This might involve a combination of self-regulation, industry standards, and government oversight, tailored to the specific risks associated with different AI applications. For example, regulations surrounding the use of AI in healthcare would differ significantly from those governing its use in marketing.
Illustrative Examples of AI and Data Interaction
AI’s ability to process and interpret vast datasets is transforming numerous industries. The interaction between AI and data isn’t a passive one; rather, it’s a dynamic relationship where AI algorithms actively shape how data is understood, used, and ultimately, how decisions are made. Let’s examine some specific instances of this powerful interplay.
AI-Powered Fraud Detection in Financial Transactions
Consider a major bank processing millions of transactions daily. Traditional methods of fraud detection often rely on rule-based systems, which can be easily bypassed by sophisticated fraudsters. AI, however, can analyze vast datasets encompassing transaction history, location data, spending patterns, and even social media activity to identify anomalies indicative of fraudulent behavior. Machine learning algorithms can learn to recognize subtle patterns and relationships that humans might miss, flagging suspicious transactions for further investigation with a significantly higher accuracy rate than older systems.
The keyword “AI dala” hints at the vast potential of data in artificial intelligence. Understanding this connection requires exploring the broader field of Artificial Intelligence , which heavily relies on data analysis for learning and decision-making. Ultimately, effective use of the keyword “AI dala” depends on a solid grasp of AI fundamentals and its data-driven nature.
For instance, an AI system might detect unusual spending patterns – a sudden surge in high-value transactions from an account with a history of small purchases – and flag it as potentially fraudulent, even if it doesn’t violate any pre-defined rules. This proactive approach allows for faster intervention and minimizes financial losses.
AI-Driven Medical Diagnosis and Treatment Planning
In healthcare, AI is revolutionizing diagnostics and treatment planning. AI algorithms can analyze medical images (X-rays, CT scans, MRIs) with remarkable accuracy, identifying subtle indicators of disease that might be missed by the human eye. For example, an AI system trained on a massive dataset of radiological images can detect early signs of cancerous tumors with a high degree of precision, enabling earlier and more effective treatment.
Beyond image analysis, AI can also integrate patient data from electronic health records (EHRs), genetic information, and lifestyle factors to create personalized treatment plans. This tailored approach ensures that patients receive the most effective and efficient care possible, optimizing outcomes and reducing healthcare costs. The AI might analyze a patient’s genetic profile and medical history to predict their likelihood of developing certain diseases, prompting proactive preventative measures.
AI in Personalized Education and Learning
In the field of education, AI is transforming the learning experience through personalized learning platforms. These platforms utilize AI algorithms to analyze student performance data, identifying individual strengths and weaknesses. Based on this analysis, the AI system can tailor educational content and pacing to meet the specific needs of each student. For example, if a student struggles with a particular concept, the AI might provide additional practice exercises or suggest alternative learning resources.
This adaptive approach ensures that students receive the support they need to succeed, improving learning outcomes and fostering a more engaging learning experience. The AI can track student progress in real-time, providing teachers with valuable insights into individual student performance and identifying areas where additional support is needed. This data-driven approach allows teachers to personalize their instruction and maximize the effectiveness of their teaching strategies.
AI-Generated Data Visualization
Imagine a three-dimensional visualization of global climate change data. The Earth is represented as a sphere, with different shades of blue representing varying ocean temperatures. Warmer areas are depicted in intense shades of red and orange, while cooler regions are represented by lighter blues and greens. The visualization dynamically changes over time, showing the progression of warming temperatures across decades.
Lines connecting various points on the globe represent migration patterns of specific animal species in response to climate change. The thickness of these lines indicates the size of the migrating population. The overall effect is a visually stunning and informative representation of complex climate data, enabling viewers to grasp the magnitude and impact of global warming with unprecedented clarity.
The AI system generating this visualization automatically selects the most effective color scheme, scales the data appropriately, and chooses the most intuitive visual representation based on the data’s complexity and the intended audience.
Improving Data Accessibility for Diverse Users
A hypothetical system could incorporate several AI-powered features to improve data accessibility. First, an AI-powered translation engine could automatically translate data into multiple languages, removing language barriers for users from diverse linguistic backgrounds. Second, the system could utilize text-to-speech and speech-to-text technologies, allowing visually impaired or dyslexic users to access and interact with the data through audio interfaces. Third, the AI could automatically generate simplified explanations of complex data, ensuring that users with varying levels of technical expertise can understand the information presented.
Finally, the system could adapt its interface and data presentation to suit the specific needs of individual users, based on their cognitive abilities and preferences.
Benefits and Drawbacks of AI-Driven Data Analysis in Healthcare
Before listing the benefits and drawbacks, it’s important to note that AI in healthcare is a rapidly evolving field, and the impact of AI-driven data analysis will continue to evolve.
- Benefits: Improved diagnostic accuracy, personalized treatment plans, efficient drug discovery, early disease detection, reduced healthcare costs, enhanced patient monitoring.
- Drawbacks: Data privacy concerns, algorithmic bias, lack of transparency in decision-making, high implementation costs, potential for job displacement, ethical considerations regarding data ownership and usage.
Conclusive Thoughts
In conclusion, the exploration of AI dala reveals a powerful synergy between artificial intelligence and data. While AI offers unprecedented opportunities for efficient data management and insightful analysis, ethical considerations and responsible development are paramount. As AI continues to advance, its impact on data management strategies, societal structures, and regulatory frameworks will only intensify. Navigating this complex landscape requires a proactive approach, balancing innovation with ethical responsibility to ensure a future where AI and data serve humanity’s best interests.
General Inquiries
What does “AI dala” actually mean?
The meaning of “AI dala” is context-dependent. It could refer to data processed by AI, AI-generated data, or even a specific AI system or project. Further clarification is needed based on the specific usage.
What are some common AI algorithms used for data processing?
Common algorithms include machine learning (e.g., linear regression, decision trees, neural networks), deep learning (e.g., convolutional neural networks, recurrent neural networks), and natural language processing techniques.
How can AI improve data accessibility for diverse users?
AI can personalize data presentation, translate languages, provide alternative data formats (audio, visual), and automate data entry and retrieval processes, making data more accessible to users with varying needs and abilities.
What are the biggest risks associated with AI data analysis?
Significant risks include biased algorithms leading to unfair outcomes, data breaches compromising privacy, and the potential for misuse of AI-driven insights for malicious purposes.